Beginner's Guide to Harnessing the Power and Ease of PySpark

4.4 out of 5
Language | : | English |
File size | : | 7247 KB |
Text-to-Speech | : | Enabled |
Screen Reader | : | Supported |
Enhanced typesetting | : | Enabled |
Print length | : | 322 pages |
Welcome to the ultimate guide for beginners who want to master the power and ease of PySpark. PySpark is the Python API for Apache Spark, a popular open-source framework for distributed data processing. With PySpark, you can harness the capabilities of Spark to perform complex data analytics tasks with ease.
This comprehensive guide is designed to take you from a complete beginner to a confident PySpark user. Through hands-on examples and clear explanations, you'll learn how to:
* Install and set up PySpark * Ingest and process data from various sources * Perform data cleaning, transformation, and manipulation * Build and train machine learning models * Visualize and analyze your results
What is PySpark?
PySpark is a powerful Python API that allows you to interact with Apache Spark, a unified analytics engine for large-scale data processing. Spark provides a distributed computing framework that enables you to:
* Process massive datasets efficiently using a cluster of computers * Perform complex data transformations and aggregations * Build machine learning models on large datasets * Analyze and visualize your data with interactive tools
PySpark makes it easy to leverage the power of Spark by providing a Python-friendly interface. With PySpark, you can write code that is both concise and efficient, making it a popular choice for data scientists, analysts, and developers.
Getting Started with PySpark
To get started with PySpark, you will need to:
1. Install Python 3.6 or later 2. Install Apache Spark 3. Install PySpark
Detailed instructions on how to install these components can be found in the official PySpark documentation.
Once you have installed PySpark, you can start using it in your Python scripts. Let's take a look at a simple example:
python import pyspark
# Create a SparkContext sc = pyspark.SparkContext()
# Create a DataFrame df = sc.parallelize([("Alice", 25),("Bob", 30),("Charlie", 28)]).toDF(["name", "age"])
# Print the DataFrame df.show()
This code creates a SparkContext, which is the entry point for interacting with Spark. It then creates a DataFrame, which is a distributed collection of data organized into named columns. In this example, the DataFrame contains three rows with two columns: "name" and "age". The show() method is used to print the contents of the DataFrame.
Data Ingestion and Processing
One of the key strengths of PySpark is its ability to ingest and process data from various sources. You can read data from files, databases, streaming sources, and more. Once you have ingested your data into PySpark, you can perform a wide range of operations on it, including:
* Cleaning and transforming data * Filtering and sorting data * Aggregating and summarizing data * Joining and merging data * Bucketing and partitioning data
PySpark provides a rich set of functions and operators that make it easy to perform these operations. For example, the following code shows how to read data from a CSV file and clean it up:
python df = spark.read.csv("data.csv", header=True, inferSchema=True)
# Drop any rows with missing data df = df.dropna()
# Convert the "age" column to an integer df = df.withColumn("age", df["age"].cast("int"))
In this example, we read the data from a CSV file using the read.csv() method. We then use the dropna() method to remove any rows that contain missing data. Finally, we use the withColumn() method to convert the "age" column from a string to an integer.
Machine Learning with PySpark
PySpark is not only great for data processing, but it also provides powerful capabilities for machine learning. With PySpark, you can build and train machine learning models on large datasets. PySpark includes a variety of machine learning algorithms, including:
* Linear regression * Logistic regression * Decision trees * Random forests * Gradient-boosted trees * Clustering algorithms
The following code shows how to build and train a simple linear regression model using PySpark:
python from pyspark.ml.regression import LinearRegression
# Create a LinearRegression object lr = LinearRegression()
# Fit the model to the data model = lr.fit(df)
# Make predictions predictions = model.transform(df)
In this example, we create a LinearRegression object and fit it to the DataFrame df. The fit() method takes the DataFrame as input and returns a fitted model object. We can then use the model to make predictions on new data.
Data Visualization and Analysis
Once you have processed and analyzed your data, you need to be able to visualize and analyze it in Free Download to gain insights. PySpark provides several tools for data visualization and analysis, including:
* Histograms * Scatter plots * Line charts * Bar charts * Pie charts * 3D visualizations
The following code shows how to create a simple histogram using PySpark:
python import matplotlib.pyplot as plt
# Create a histogram df["age"].hist()
# Show the plot plt.show()
In this example, we create a histogram of the "age" column in the DataFrame df. The hist() method takes the column as input and generates a histogram. We then use the show() method to display the plot.
PySpark is a powerful tool for big data analytics and machine learning. It provides a Python-friendly interface to Apache Spark, making it easy to process and analyze large datasets. With PySpark, you can quickly and efficiently perform complex data operations, build and train machine learning models, and visualize your results.
This beginner's guide has provided you with a solid foundation for using PySpark. As you continue to explore PySpark, you will discover even more powerful capabilities that can help you unlock the full potential of your data.
4.4 out of 5
Language | : | English |
File size | : | 7247 KB |
Text-to-Speech | : | Enabled |
Screen Reader | : | Supported |
Enhanced typesetting | : | Enabled |
Print length | : | 322 pages |
Do you want to contribute by writing guest posts on this blog?
Please contact us and send us a resume of previous articles that you have written.
Book
Novel
Page
Chapter
Text
Story
Genre
Reader
Library
Paperback
E-book
Magazine
Newspaper
Paragraph
Sentence
Bookmark
Shelf
Glossary
Bibliography
Foreword
Preface
Synopsis
Annotation
Footnote
Manuscript
Scroll
Codex
Tome
Bestseller
Classics
Library card
Narrative
Biography
Autobiography
Memoir
Reference
Encyclopedia
Victoria Lindstrom
Travis Heermann
Stacey Duckett
Simon Scarrow
Suzi Livingstone
William Wood
Susan B
Stacy Claflin
Selene Yeager
Stephen Beaumont
Steven Eisenberg
Worth Books
Justin Darcy
Scott Pritchard
Unrulynerdgirl Bear
Vicki Lansky
Sophie Allison
The 12 Step Support Companion
Steven Shomler
Stephen Blauer
Light bulbAdvertise smarter! Our strategic ad space ensures maximum exposure. Reserve your spot today!
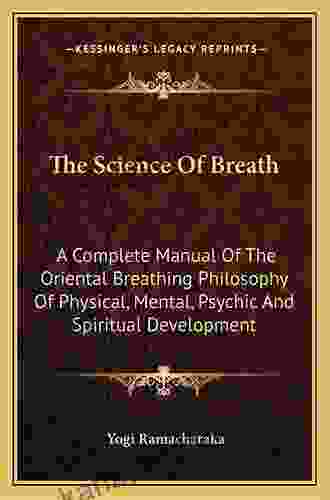

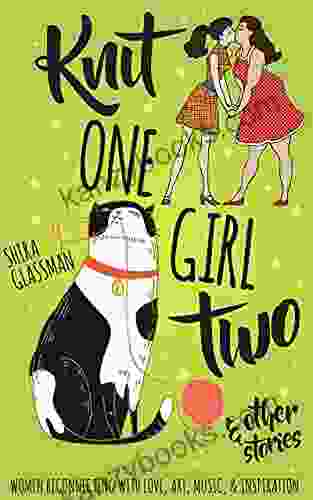

- Philip BellFollow ·3.7k
- José SaramagoFollow ·7.9k
- Nick TurnerFollow ·17.8k
- Todd TurnerFollow ·16.4k
- Ernest HemingwayFollow ·7.8k
- Kelly BlairFollow ·18.3k
- Alec HayesFollow ·18.2k
- Jayson PowellFollow ·12.1k
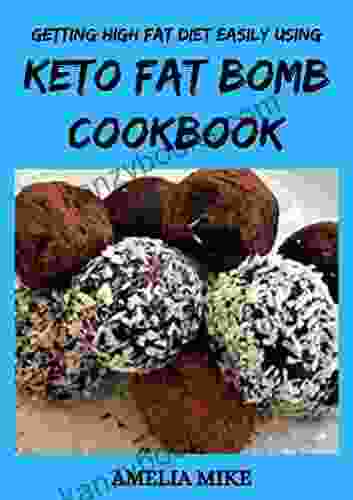

Getting High Fat Diet Easily Using Keto Fat Bomb Cookbook
Unveiling the Power of Fat...
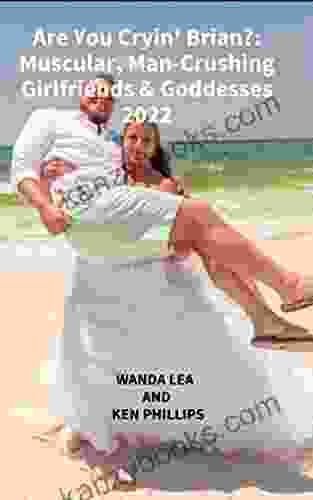

Are You Cryin' Brian? Find the Inspiration and Humor in...
Life can be full of...
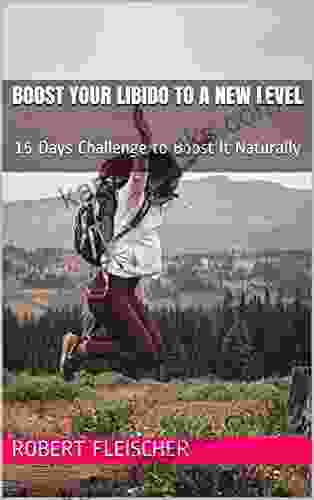

Unlock Your Vitality: The 15-Day Natural Energy Boost...
Are You Ready to...
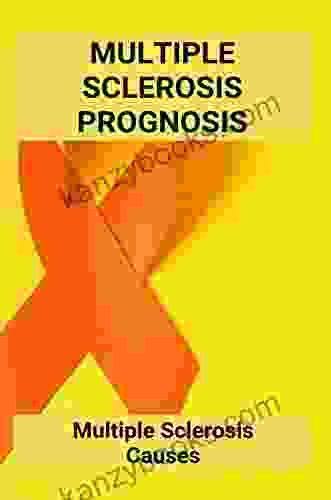

Multiple Sclerosis Life Expectancy: Unveiling the Impact...
Multiple Sclerosis (MS) is a...
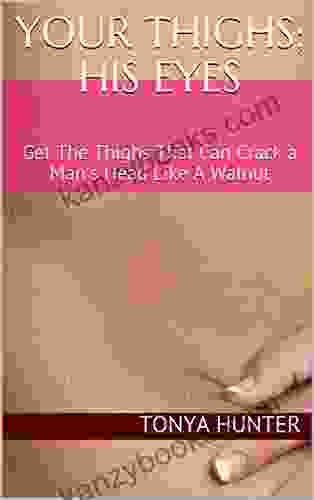

Get The Thighs That Can Crack Man Head Like Walnut
Are you tired of weak, flabby...
4.4 out of 5
Language | : | English |
File size | : | 7247 KB |
Text-to-Speech | : | Enabled |
Screen Reader | : | Supported |
Enhanced typesetting | : | Enabled |
Print length | : | 322 pages |